AI Insurance might be more important than you think
Two of our board members are veterans in both AI and Insurance.
A topic often not appreciated by the public and sometimes by regulators themselves is the power of the insurance industry to create helpful or harmful defacto regulation.
For the last two years we have been researching the application of Errors and Omissions or "Cyber Liability" insurance - a kind of liability insurance that most companies interacting with financial data, governments, and large corporate partners must have — to include and exclude AI uses.
The insurance industry is slow to respond but the stakes are high. The industry must through its operation learn how to sort out mundane from high risk use cases of AI, and decide what transparency and visibility will allow them to achieve that in an accurate rather than a superficial way. If this is done poorly, then we will create a moral hazard, where mundane actors subsidize un-careful risk takers, and if done properly we have a functional market driven regulatory intervention that will likely outperform legislative regulators and be less open to regulatory capture.
As a demonstration that the state of affairs has not been solve, both Microsoft and Google have taken the extraordinary step of indemnifying their own cloud computing users against copyright infringement claims for using AI, in order to drive business rather than wait for the insurance industry to create products.
You will hopefully hear more from our board on this topic soon.
In the mean time, here are some papers you may find of interest.
Some from friends and community members, faculty and former students from our ML Alignment and Theory Scholars, whom we provided a small grant.
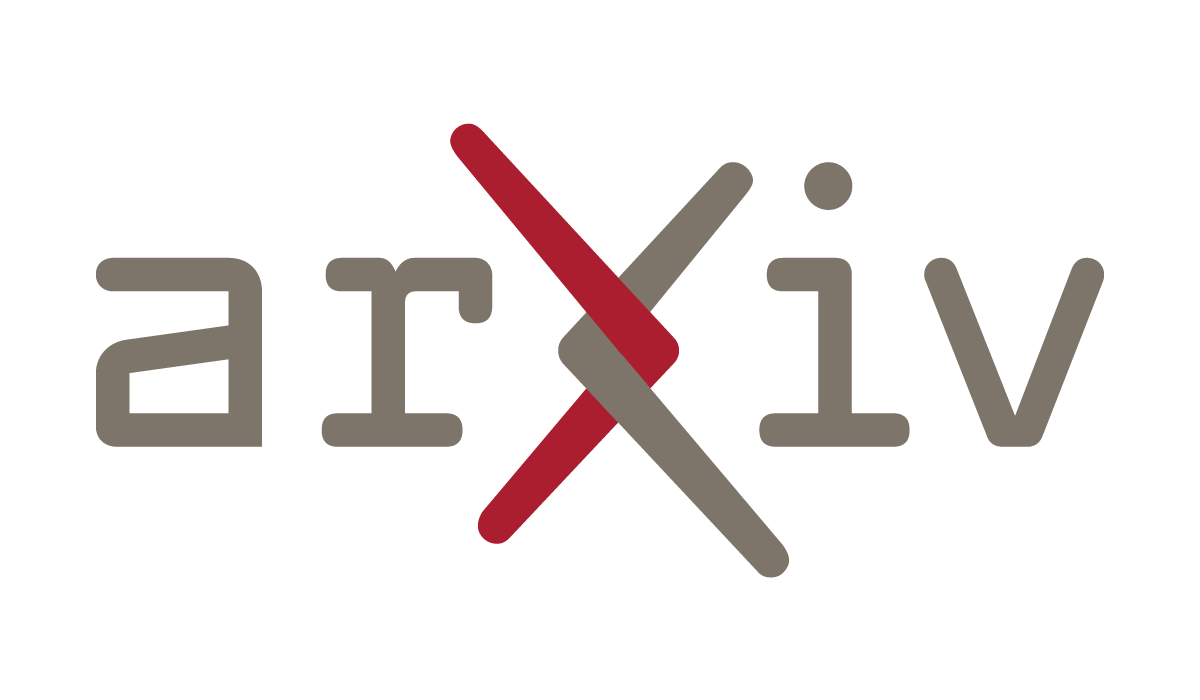
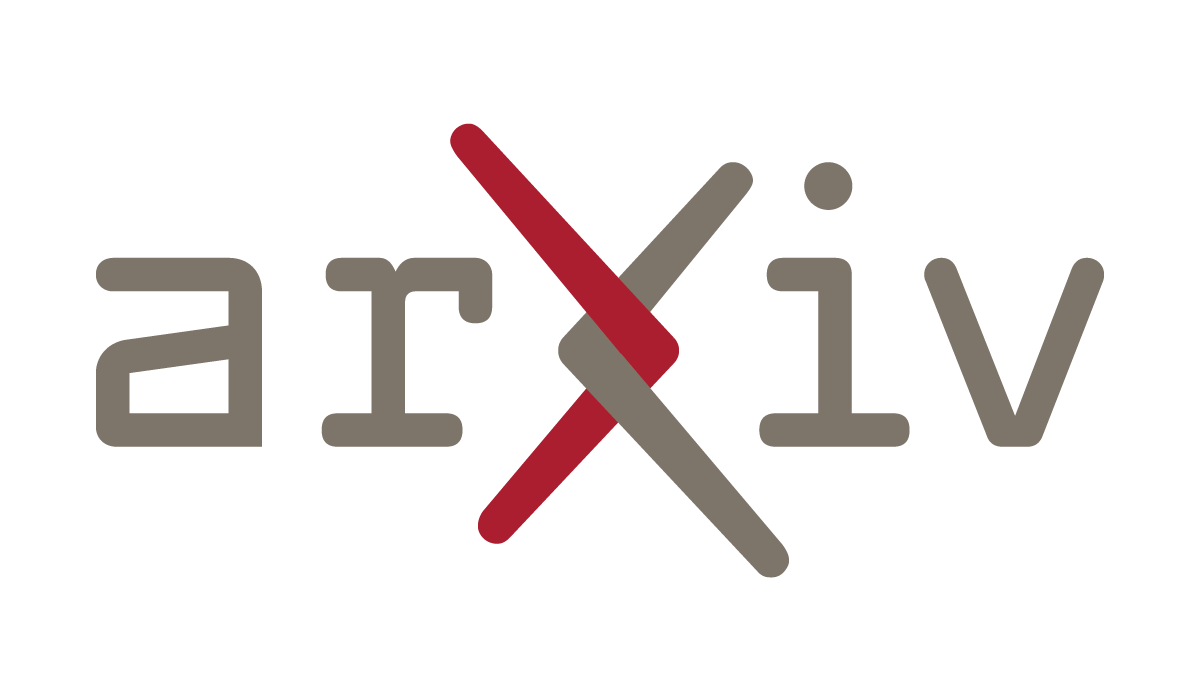
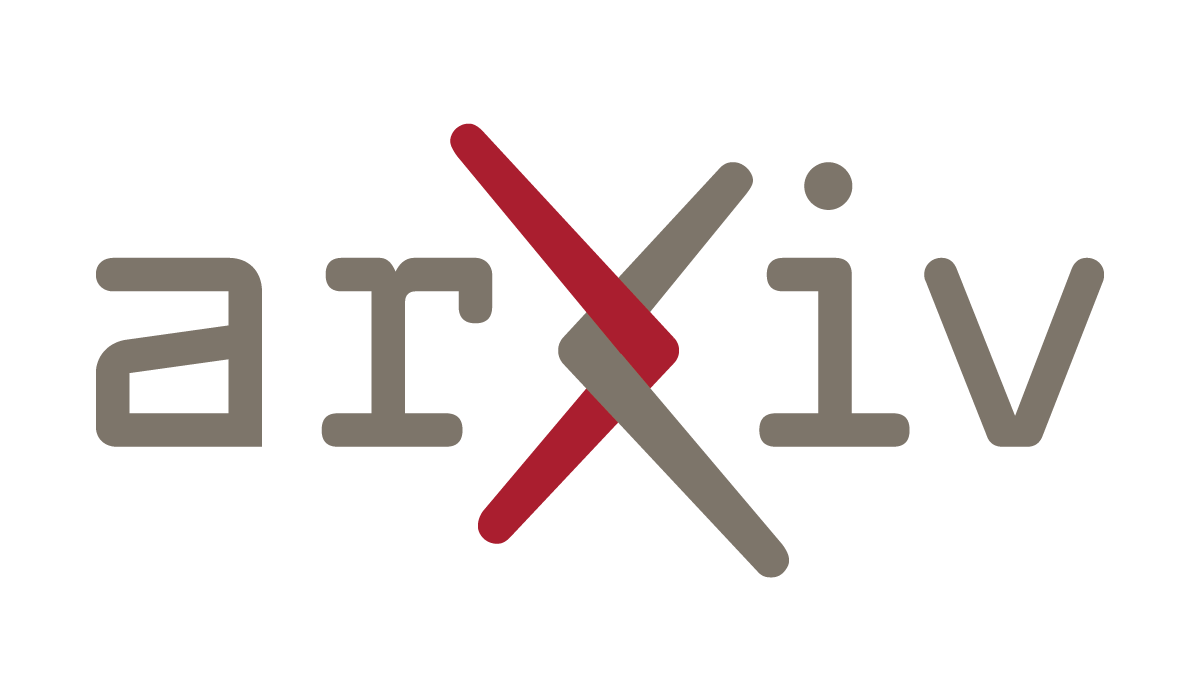